Real-time Facial Palsy with Age and Gender Detection
DOI:
https://doi.org/10.51173/eetj.v1i1.10Keywords:
Facial Palsy, Face Detection, Computer Vision, Machine LearningAbstract
Facial palsy (FP) is a disease that affects the facial nerves, leading to deviation of the face towards the opposite direction of the injury, with an inability to control facial movements. Diagnosis is typically based on the clinician's judgment, considering the patient's age, gender, and treatment type. However, this method is prone to errors due to doctors' exposure to fatigue and other problems. Therefore, the use of computer vision (CV) systems to automatically detect FP has become crucial. Deep learning is a promising candidate for accurate and cost-effective FP detection. In this context, this work proposes a real-time system that uses a deep learning (DL) algorithm to detect FP, age, and gender. The proposed system could be used by patients at home or as a diagnostic tool for doctors. The proposed system achieves an accuracy of 98% by using datasets containing 19,239 normal images, 834 left palsy images, and 801 right palsy images.
References
Barbosa, J.; Lee, K.; Lee, S.; Lodhi, B.; Cho, J.-G.; Seo, W.-K.; Kang, J. Efficient quantitative assessment of facial paralysis using iris segmentation and active contour-based key points detection with hybrid classifier. BMC medical imaging 2016, 16, 1-18. https://link.springer.com/article/10.1186/s12880-016-0117-0
Baugh, R.F.; Basura, G.J.; Ishii, L.E.; Schwartz, S.R.; Drumheller, C.M.; Burkholder, R.; Deckard, N.A.; Dawson, C.; Driscoll, C.; Gillespie, M.B. Clinical practice guideline: Bell’s palsy. Otolaryngology–Head and Neck Surgery 2013, 149, S1-S27. https://journals.sagepub.com/doi/full/10.1177/0194599813505967
Szczepura, A.; Holliday, N.; Neville, C.; Johnson, K.; Khan, A.J.K.; Oxford, S.W.; Nduka, C. Raising the digital profile of facial palsy: national surveys of patients’ and clinicians’ experiences of changing UK treatment pathways and views on the future role of digital technology. Journal of medical Internet research 2020, 22, e20406.
https://www.jmir.org/2020/10/e20406/
Ahmed, A. When is facial paralysis Bell palsy? Current diagnosis and treatment. Cleve Clin J Med 2005, 72, 398-401. https://doi.org/10.3949/ccjm.72.5.398
Movahedian, B.; Ghafoornia, M.; Saadatnia, M.; Falahzadeh, A.; Fateh, A. Epidemiology of Bell’s palsy in Isfahan, Iran. Neurosciences Journal 2009, 14, 186-187.
https://pesquisa.bvsalud.org/portal/resource/pt/emr-92261
Liu, X.; Wang, Y.; Luan, J. Facial paralysis detection in infrared thermal images using asymmetry analysis of temperature and texture features. Diagnostics 2021, 11, 2309.
https://doi.org/10.3390/diagnostics11122309
Xia, Y.; Nduka, C.; Kannan, R.Y.; Pescarini, E.; Berner, J.E.; Yu, H. AFLFP: A database with annotated facial landmarks for facial palsy. IEEE Transactions on Computational Social Systems 2022, 10, 1975-1985.
DOI: 10.1109/TCSS.2022.3187622
Haase, D.; Minnigerode, L.; Volk, G.F.; Denzler, J.; Guntinas-Lichius, O. Automated and objective action coding of facial expressions in patients with acute facial palsy. European Archives of Oto-Rhino-Laryngology 2015, 272, 1259-1267. https://link.springer.com/article/10.1007/s00405-014-3385-8
Dong, J.; Ma, L.; Li, Q.; Wang, S.; Liu, L.-a.; Lin, Y.; Jian, M. An approach for quantitative evaluation of the degree of facial paralysis based on salient point detection. In Proceedings of the 2008 International Symposium on Intelligent Information Technology Application Workshops, 2008; pp. 483-486.
DOI: 10.1109/IITA.Workshops.2008.93
Kim, J.; Jeong, H.; Cho, J.; Pak, C.; Oh, T.S.; Hong, J.P.; Kwon, S.; Yoo, J. Numerical approach to facial palsy using a novel registration method with 3D facial landmark. Sensors 2022, 22, 6636.
https://doi.org/10.3390/s22176636
Azoulay, O.; Ater, Y.; Gersi, L.; Glassner, Y.; Bryt, O.; Halperin, D. Mobile application for diagnosis of facial palsy. In Proceedings of the Proc. 2nd Int. Conf. Mobile Inf. Technol. Med, 2014; pp. 1-4.
Barbosa, J.; Lee, K.; Lee, S.; Lodhi, B.; Cho, J.-G.; Seo, W.-K.; Kang, J. Efficient quantitative assessment of facial paralysis using iris segmentation and active contour-based key points detection with hybrid classifier. BMC medical imaging 2016, 16, 1-18. https://link.springer.com/article/10.1186/s12880-016-0117-0
Codari, M.; Pucciarelli, V.; Stangoni, F.; Zago, M.; Tarabbia, F.; Biglioli, F.; Sforza, C. Facial thirds–based evaluation of facial asymmetry using stereophotogrammetric devices: Application to facial palsy subjects. Journal of Cranio-Maxillofacial Surgery 2017, 45, 76-81.
https://doi.org/10.1016/j.jcms.2016.11.003
Storey, G.; Jiang, R.; Bouridane, A. Role for 2D image generated 3D face models in the rehabilitation of facial palsy. Healthcare technology letters 2017, 4, 145-148.
https://doi.org/10.1049/htl.2017.0023
Storey, G.; Jiang, R.; Keogh, S.; Bouridane, A.; Li, C.-T. 3DPalsyNet: A facial palsy grading and motion recognition framework using fully 3D convolutional neural networks. IEEE access 2019, 7, 121655-121664.
DOI: 10.1109/ACCESS.2019.2937285
Wang, T.; Zhang, S.; Liu, L.A.; Wu, G.; Dong, J. Automatic facial paralysis evaluation augmented by a cascaded encoder network structure. IEEE Access 2019, 7, 135621-135631.
DOI: 10.1109/ACCESS.2019.2942143
Barbosa, J.; Seo, W.-K.; Kang, J. paraFaceTest: an ensemble of regression tree-based facial features extraction for efficient facial paralysis classification. BMC Medical Imaging 2019, 19, 1-14.
https://link.springer.com/article/10.1186/s12880-019-0330-8
Jiang, C.; Wu, J.; Zhong, W.; Wei, M.; Tong, J.; Yu, H.; Wang, L. Automatic facial paralysis assessment via computational image analysis. Journal of Healthcare Engineering 2020, 2020, 2398542.
https://doi.org/10.1155/2020/2398542
Barrios Dell'Olio, G.; Sra, M. Farapy: An augmented reality feedback system for facial paralysis using action unit intensity estimation. In Proceedings of the The 34th Annual ACM Symposium on User Interface Software and Technology, 2021; pp. 1027-1038. https://doi.org/10.1145/3472749.3474803
Nguyen, D.-P.; Ho Ba Tho, M.-C.; Dao, T.-T. Enhanced facial expression recognition using 3D point sets and geometric deep learning. Medical & Biological Engineering & Computing 2021, 59, 1235-1244.
https://link.springer.com/article/10.1007/s11517-021-02383-1
Parra-Dominguez, G.S.; Sanchez-Yanez, R.E.; Garcia-Capulin, C.H. Facial paralysis detection on images using key point analysis. Applied Sciences 2021, 11, 2435.
https://doi.org/10.3390/app11052435
Chiesa-Estomba, C.M.; Echaniz, O.; Suarez, J.A.S.; González-García, J.A.; Larruscain, E.; Altuna, X.; Medela, A.; Graña, M. Machine learning models for predicting facial nerve palsy in parotid gland surgery for benign tumors. journal of surgical research 2021, 262, 57-64.
https://doi.org/10.1016/j.jss.2020.12.053
Amsalam, A.S.; Al-Naji, A.; Daeef, A.Y.; Chahl, J. Computer Vision System for Facial Palsy Detection. Journal of Techniques 2023, 5, 44-51.
https://doi.org/10.51173/jt.v5i1.1133
Amsalam, A.S.; Al-Naji, A.; Daeef, A.Y.; Chahl, J. Automatic facial palsy, age and gender detection using a raspberry pi. BioMedInformatics 2023, 3, 455-466.
https://doi.org/10.3390/biomedinformatics3020031
Amsalam, A.S.; Al-Naji, A.; Daeef, A.Y. Facial palsy detection using pre-trained deep learning models: A comparative study. In Proceedings of the AIP Conference Proceedings, 2024.
https://doi.org/10.1063/5.0209928
Chandaliya, P.K.; Kumar, V.; Harjani, M.; Nain, N. Scdae: Ethnicity and gender alteration on clf and utkface dataset. In Proceedings of the International Conference on Computer Vision and Image Processing, 2019; pp. 294-306. https://link.springer.com/chapter/10.1007/978-981-15-4018-9_27
Abayomi-Alli, O.O.; Damaševičius, R.; Maskeliūnas, R.; Misra, S. Few-shot learning with a novel voronoi tessellation-based image augmentation method for facial palsy detection. Electronics 2021, 10, 978.
https://doi.org/10.3390/electronics10080978
Afridi, T.H.; Alam, A.; Khan, M.N.; Khan, J.; Lee, Y.-K. A multimodal memes classification: A survey and open research issues. In Proceedings of the Innovations in Smart Cities Applications Volume 4: The Proceedings of the 5th International Conference on Smart City Applications, 2021; pp. 1451-1466.
https://link.springer.com/chapter/10.1007/978-3-030-66840-2_109
Codeluppi, L.; Venturelli, F.; Rossi, J.; Fasano, A.; Toschi, G.; Pacillo, F.; Cavallieri, F.; Giorgi Rossi, P.; Valzania, F. Facial palsy during the COVID‐19 pandemic. Brain and behavior 2021, 11, e01939.
https://doi.org/10.1002/brb3.1939
Ansari, S.A.; Jerripothula, K.R.; Nagpal, P.; Mittal, A. Eye-focused Detection of Bell's Palsy in Videos. arXiv preprint arXiv:2201.11479 2022.
https://doi.org/10.48550/arXiv.2201.11479
Saxena, K.; Khan, Z.; Singh, S. Diagnosis of diabetes mellitus using k nearest neighbor algorithm. International Journal of Computer Science Trends and Technology (IJCST) 2014, 2, 36-43.
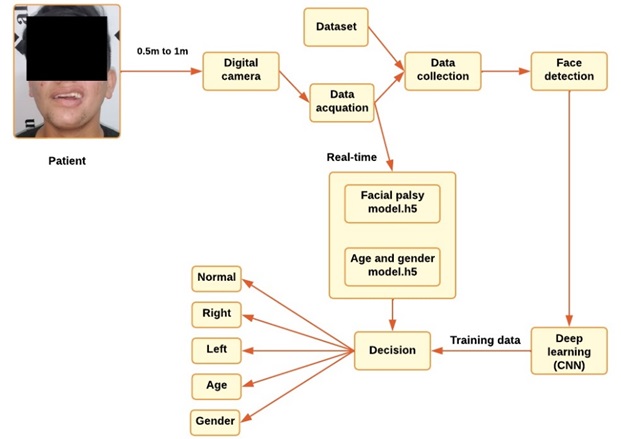